Research Overview (Non-Technical)
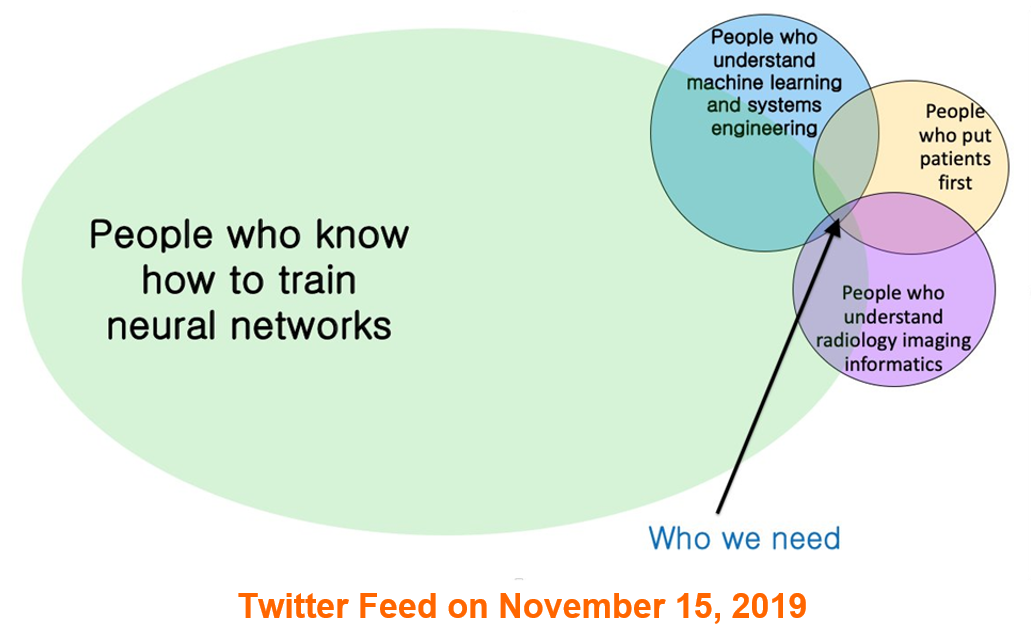
On November 15, 2019, a post appeared on Twitter answering the following question: Who do we need in healthcare today? (figure on the left hand side) Healthcare is in need of professionals (rigorously) trained in the theoretical and computational aspects of machine learning and systems engineering, radiology imaging informatics, and who hold patient care much much higher than "singular technological aspirations". This ideology is rigorously practiced in the Algorithms in Theory and Practice (ATP) Lab at LUMS. As the name of my research lab suggests, we study fundamental properties of algorithms, its computational virtues, limitations, and challenges, and its efficacy in completing a clinical task in question. Our focus is always on solving a clinical problem or completing a clinical task successfully i.e., we value effectiveness over elegance. In fact, we strive to develop a compact and explainable computational algorithm (elegance) which robustly and accurately solves a clinical problem (effective). At ATP Lab, my students and I are working on several projects in computational clinical imaging expounded below.
The RANO-AI Program

Semi-supervised Learning in Cardiac Image Analysis
ATP Lab is also pursuing research endeavors in cardiology. We are developing computational algorithms, to be integrated in a pipeline, for radiomics in cardiac imaging. The goal is robust and accurate prediction of cardiac pathologies from 3D MRI (or CT) scans only and quantification of clinical measures of interest, which define left-ventricle and right-ventricle function. We are currently working on a novel semi-supervised segmentation algorithm for multi-phase 3D cardiac image segmentation. This will be followed by an extensive radiomics study with 3D cardiac MRI (or CT) scans.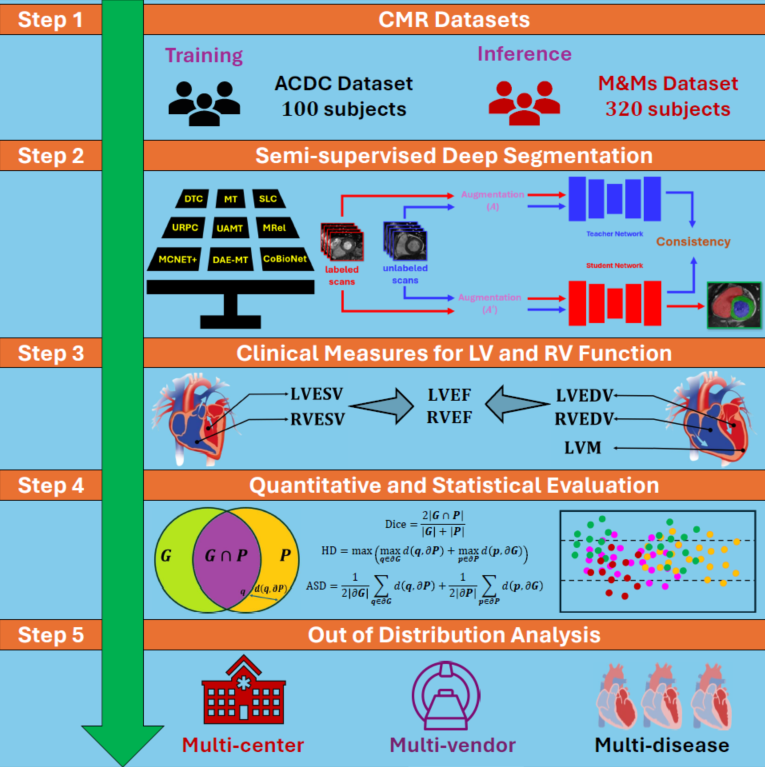